Unveiling Lesser-Known Strategies for Deploying Large Language Models on Cloud Platforms
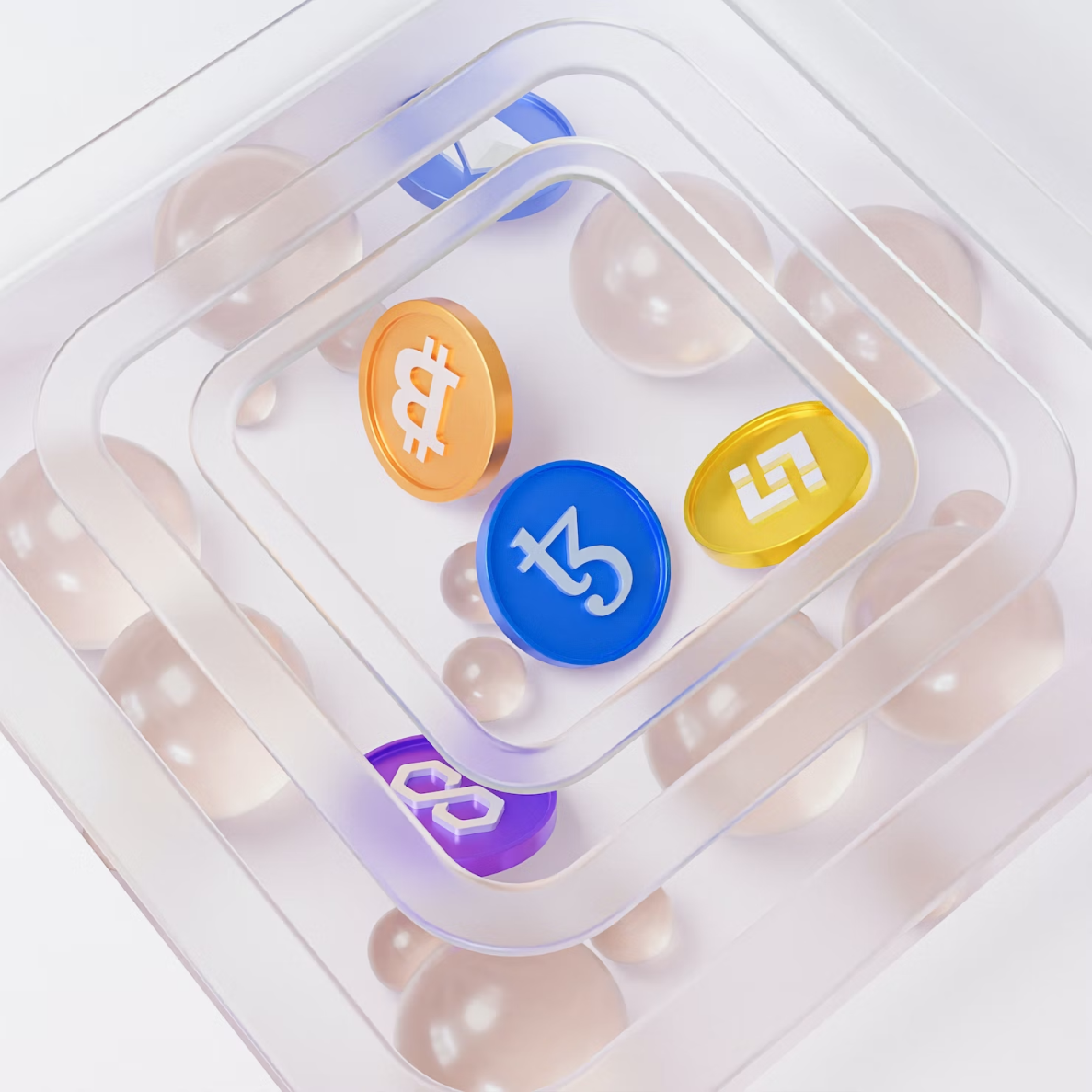
Large Language Models (LLMs) have become increasingly popular over the last year or so and are already a force to be reckoned with, reshaping and transforming the different sectors with their capabilities. Ranging from conversational AI to complex analytical ones, LLMs offer new and endless possibilities. Deploying these models to the cloud as well as to other cloud platforms is accompanied by its own set of issues and difficulties that should be paid attention to.
Taking into consideration the cost efficiency and scalability
Such features as scalability shine out when LLMs are deployed on cloud platforms, enabling them to flexibly allocate resources whenever necessary. Nevertheless, balancing the growth with the costs is a matter of priority in order to achieve an increase in the output without overspending. One of the key features of cloud services is the pay-as-you-go model, where users pay for the use of a certain resource. This makes cost management critical. The adoption of auto-scaling technology, deep scheduling, and the selection of perfectly matched instance types are proven to be efficient. Besides the ongoing management and adjustment of resources according to the usage level is also necessary to avoid over-service provision.
The deployment process of LLM is essentially about processing large volumes of data, which includes sensitive or proprietary information. The risk of data exposure is introduced by public clouds through multitenancy which is the case of sharing of the same physical hardware amongst multiple instances. While the cloud providers stress the security measures, but the risk mitigation is vital. The selection of providers with tight security standards and also additional security measures such as encryption and authorization are significant steps to secure patients’ data.
Managing stateful model deployment
LLMs are generally considered stateful which means they will retain information over a series of conversations for the purpose of learning. On the other hand, the problem of the stateful nature of these models introduces difficulties when managing them in cloud environments. Orchestration tools like Kubernetes can offer support for stateful deployments by provisioning persistent storage options. Maintaining the state of LLMs requires a careful configuration of these tools to ensure session continuity and higher performance.
With the surge in the demand for generative AI, cloud platform deployment by many corporations has become the primary method of implementing LLMs for most companies. Although the convenience of up push of the cloud approach is unarguable, it is necessary to consider its pitfalls as well to ensure the efficiency of the project and the amount of money invested. Through the use of lesser-known strategies and staying guarded, companies can fully exploit the advantages of artificially intelligent technology without its hazards.
There is a need to point out that using large language models on cloud computing results in unequaled possibilities for creativity and expansion all over sectors. Though these involve considerable cost control, data privacy, and stateful model deployment, they are still able to assist the successful implementation of the system. Given adequate risk management strategies in place, organizations can leverage LLMs while avoiding pitfalls.
The article originally story appeared on infoworld.com.
Unveiling Lesser-Known Strategies for Deploying Large Language Models on Cloud Platforms
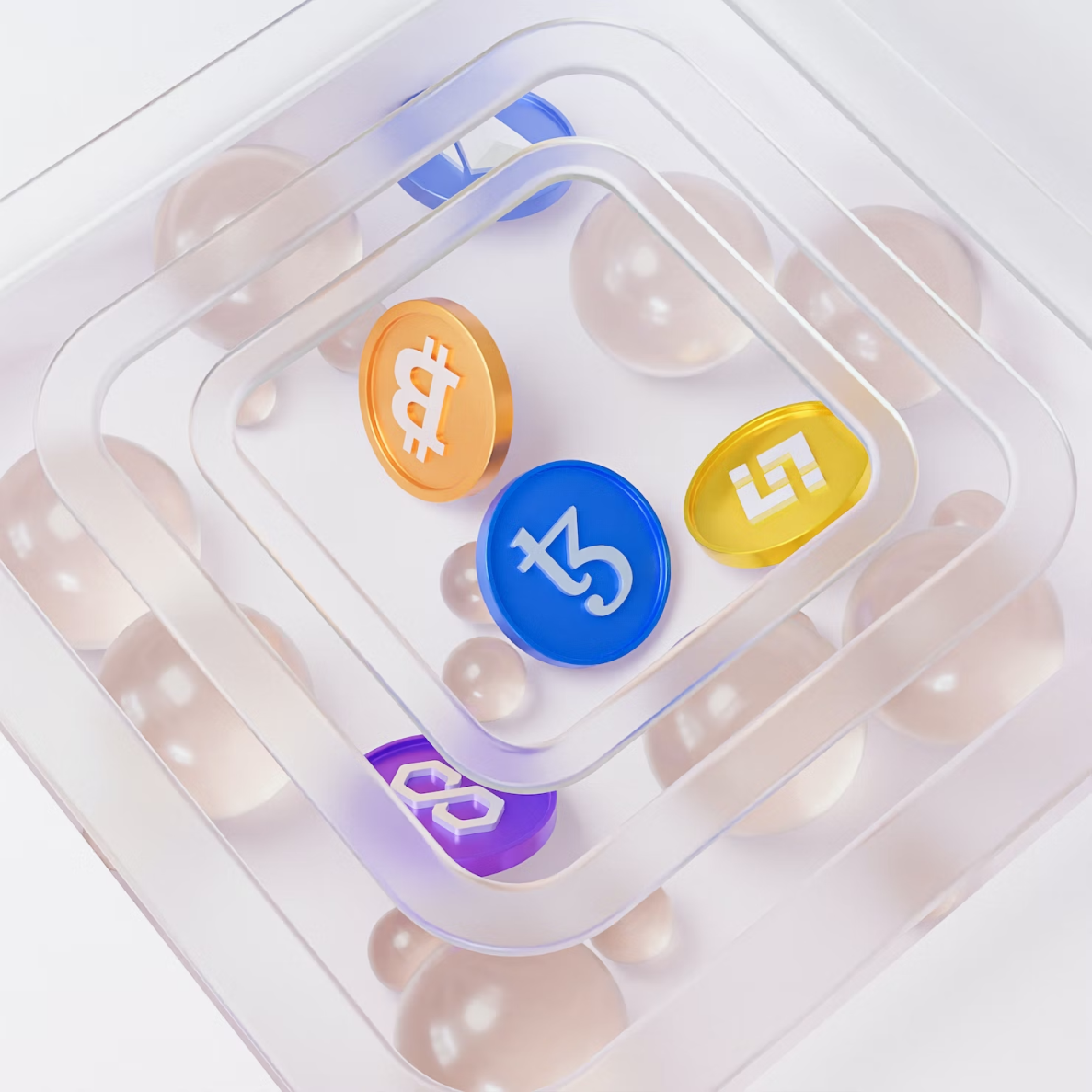
Large Language Models (LLMs) have become increasingly popular over the last year or so and are already a force to be reckoned with, reshaping and transforming the different sectors with their capabilities. Ranging from conversational AI to complex analytical ones, LLMs offer new and endless possibilities. Deploying these models to the cloud as well as to other cloud platforms is accompanied by its own set of issues and difficulties that should be paid attention to.
Taking into consideration the cost efficiency and scalability
Such features as scalability shine out when LLMs are deployed on cloud platforms, enabling them to flexibly allocate resources whenever necessary. Nevertheless, balancing the growth with the costs is a matter of priority in order to achieve an increase in the output without overspending. One of the key features of cloud services is the pay-as-you-go model, where users pay for the use of a certain resource. This makes cost management critical. The adoption of auto-scaling technology, deep scheduling, and the selection of perfectly matched instance types are proven to be efficient. Besides the ongoing management and adjustment of resources according to the usage level is also necessary to avoid over-service provision.
The deployment process of LLM is essentially about processing large volumes of data, which includes sensitive or proprietary information. The risk of data exposure is introduced by public clouds through multitenancy which is the case of sharing of the same physical hardware amongst multiple instances. While the cloud providers stress the security measures, but the risk mitigation is vital. The selection of providers with tight security standards and also additional security measures such as encryption and authorization are significant steps to secure patients’ data.
Managing stateful model deployment
LLMs are generally considered stateful which means they will retain information over a series of conversations for the purpose of learning. On the other hand, the problem of the stateful nature of these models introduces difficulties when managing them in cloud environments. Orchestration tools like Kubernetes can offer support for stateful deployments by provisioning persistent storage options. Maintaining the state of LLMs requires a careful configuration of these tools to ensure session continuity and higher performance.
With the surge in the demand for generative AI, cloud platform deployment by many corporations has become the primary method of implementing LLMs for most companies. Although the convenience of up push of the cloud approach is unarguable, it is necessary to consider its pitfalls as well to ensure the efficiency of the project and the amount of money invested. Through the use of lesser-known strategies and staying guarded, companies can fully exploit the advantages of artificially intelligent technology without its hazards.
There is a need to point out that using large language models on cloud computing results in unequaled possibilities for creativity and expansion all over sectors. Though these involve considerable cost control, data privacy, and stateful model deployment, they are still able to assist the successful implementation of the system. Given adequate risk management strategies in place, organizations can leverage LLMs while avoiding pitfalls.
The article originally story appeared on infoworld.com.