How AI Diagnosis and Treatment Applications Boost the Quality of Healthcare – Report
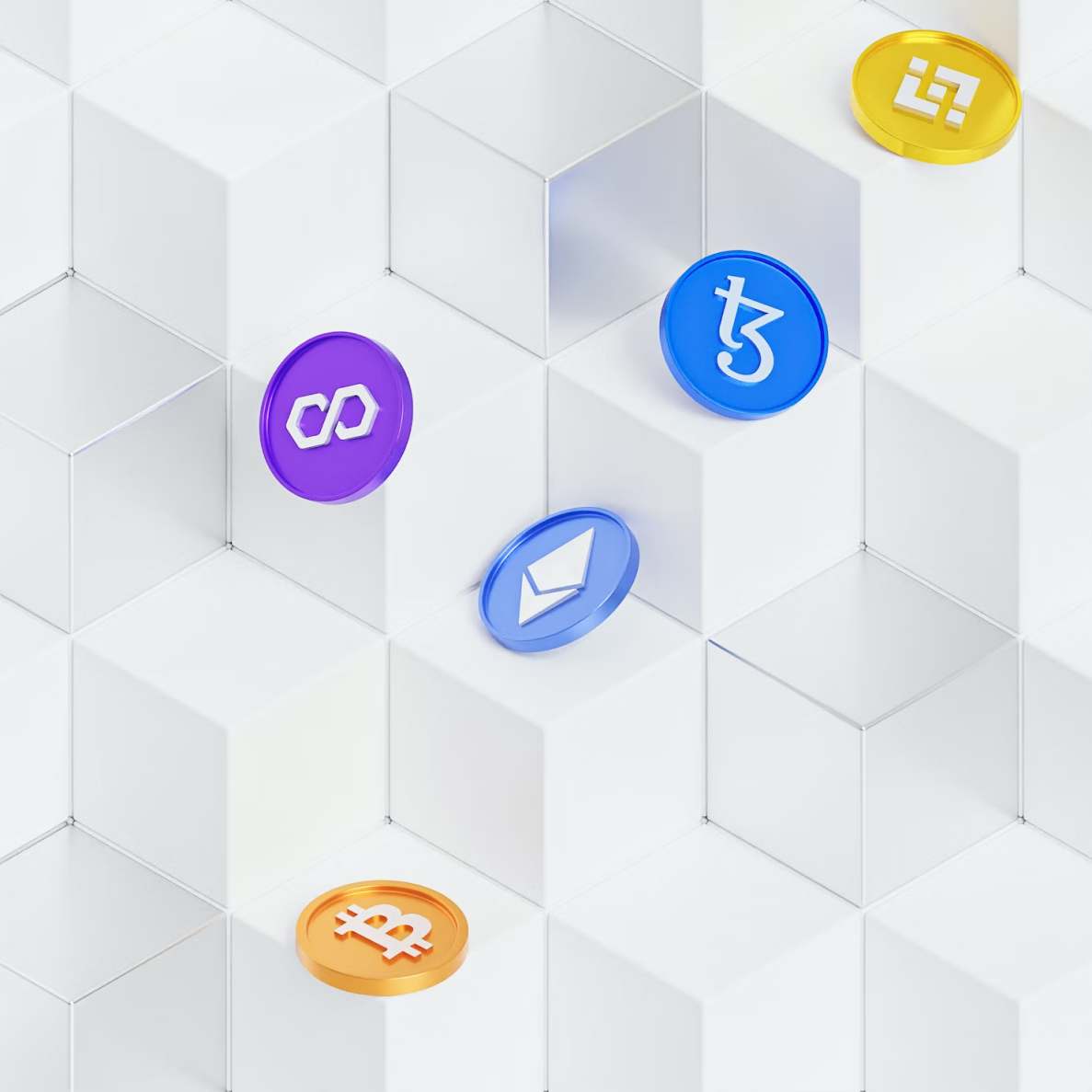
In recent years, the intersection of artificial intelligence (AI) and healthcare has become an area of intense research and transformative potential. AI diagnosis, which uses a wide range of technologies capable of performing tasks that typically require human intelligence, is pivotal in reshaping various treatment methods. This article aims to explore the multifaceted applications of AI in healthcare, mainly focusing on its contributions to diagnosing diseases and formulating treatment plans.
The evolution of AI in medical contexts has been both rapid and revolutionary. From its nascent stages of simple data analysis to its current capabilities in complex decision-making, AI has increasingly become an integral component of modern healthcare systems. Its integration into medical practices has significantly improved patient care’s accuracy, efficiency, and personalization.
Background
The journey of AI in healthcare began in the late 20th century, marked by pioneering efforts to integrate computational power into medical practices. The earliest applications were rudimentary by today’s standards, primarily focused on database management and basic patient record systems. In the 1960s and 70s, more sophisticated systems like the Dendral project, which interpreted organic chemical mass spectra, and MYCIN, developed at Stanford University for diagnosing blood infections and recommending antibiotics, laid the groundwork for AI in medical diagnostics. These systems used rule-based methods to mimic human decision-making, offering early glimpses into AI’s potential in healthcare.
Milestones in AI Development for Healthcare Purposes
- Rule-Based Expert Systems: In the 1980s, expert systems like CADUCEUS and INTERNIST-I demonstrated AI’s potential in capturing medical knowledge and diagnostic reasoning. Though limited by the technology of their time, these systems were significant in showcasing how AI could store and utilize vast medical knowledge.
- Advent of Machine Learning: The 1990s and 2000s witnessed a pivotal shift with the introduction of machine learning (ML). Algorithms could now learn from data, improving their accuracy and utility over time. This era saw the development of neural networks and decision trees, crucial in analyzing complex medical data.
- Genomics and Personalized Medicine: Completing the Human Genome Project in the early 2000s opened new avenues for AI in genomics. AI’s ability to analyze large genetic datasets has been instrumental in the growth of personalized medicine, tailoring healthcare based on individual genetic profiles.
- Deep Learning Revolution: The 2010s marked the deep learning revolution, characterized by the advent of algorithms capable of feature learning from large datasets. This breakthrough led to significant advancements in medical imaging, drug discovery, and predictive analytics.
Integration of AI with Traditional Medical Practices
The integration of AI into healthcare has required close collaboration with medical professionals. AI systems complement the expertise of doctors, nurses, and other healthcare workers. This coordination is crucial for fine-tuning AI applications for practical, real-world medical scenarios.
Alongside technological advancements, there has been a growing emphasis on developing ethical and regulatory frameworks to govern the use of AI in healthcare. These frameworks are essential to ensure patient safety, data privacy, and the responsible use of AI.
The integration process also involves educating and training healthcare professionals in AI technologies. This education ensures medical practitioners can work alongside AI tools, understanding their capabilities and limitations.
AI has played a significant role in enhancing patient engagement and the rise of telemedicine. Tools such as chatbots for preliminary diagnosis and mobile apps for health monitoring have brought healthcare closer to patients, making it more accessible and personalized.
AI in Diagnosis
Image-based Diagnostics
- Radiology and Imaging Analysis:
AI has revolutionized radiology by enhancing the interpretation of medical images such as X-rays, CT scans, and MRIs. Machine learning algorithms, especially deep learning models, can now accurately detect anomalies like tumors, fractures, and other pathological changes. For instance, AI systems can identify patterns in lung imaging indicative of diseases such as pneumonia or tuberculosis, often at early stages that may be challenging for the human eye to discern.
- Dermatology and Skin Disease Recognition:
In dermatology, AI algorithms have shown proficiency in recognizing skin lesions and differentiating between benign and malignant conditions. Utilizing vast databases of skin images, these systems help dermatologists detect skin cancers like melanoma early, facilitating timely treatment. AI tools can also assist patients in monitoring skin changes, allowing for early consultation and diagnosis.
- Ophthalmology and Retinal Image Interpretation:
AI has made significant strides in ophthalmology, particularly in analyzing retinal images. Algorithms have been developed to detect diabetic retinopathy, glaucoma, and age-related macular degeneration, among the leading causes of blindness. These AI systems provide eye care professionals with powerful tools to identify and treat these conditions much earlier than possible.
Genetic and Laboratory Data Analysis
- Predictive Modeling in Genomics:
AI’s role in genomics involves analyzing vast genetic data sets to predict disease risk and treatment outcomes. By employing techniques like machine learning, AI can identify genetic markers associated with diseases, thereby aiding in early detection and personalized treatment plans. This approach is particularly impactful in oncogenetics, where AI helps understand the genetic basis of cancers.
- Automation in Laboratory Result Interpretation:
AI has automated and improved the accuracy of laboratory result interpretation. Algorithms analyze blood tests, urinalysis, and other laboratory data, providing quicker and more accurate diagnoses. This automation reduces the workload on laboratory personnel and minimizes human error, leading to better patient outcomes.
AI in Diagnostic Decision Support
- Case Studies of AI-assisted Diagnostics:
Various case studies demonstrate the successful implementation of AI in diagnostic processes. For example, hospitals have employed AI systems to diagnose neurological disorders, cardiovascular diseases, and various cancers, often revealing subtleties missed in traditional analyses.
- Improving Accuracy and Reducing Diagnostic Errors:
AI contributes significantly to reducing diagnostic errors, a significant healthcare concern. By providing a second, unbiased opinion, AI systems can identify discrepancies in diagnoses and suggest alternative possibilities. This dual-check system ensures accuracy and can be life-saving in critical care and emergency medicine.
- Ethical Considerations and Limitations:
Despite the benefits, the use of AI in diagnosis raises ethical questions, particularly regarding data privacy, consent, and the potential for algorithmic bias. Ensuring that AI systems are from diverse datasets is crucial to avoid biases that could lead to misdiagnosis. Furthermore, the reliance on AI should not diminish the role of human judgment in healthcare; instead, we should view AI as a tool that augments the expertise of medical professionals.
AI in Treatment Planning and Management
Personalized Medicine
- Tailoring Treatment Based on Genetic Information:
AI’s role in personalized medicine is transformative, especially in utilizing genetic information to tailor treatments to individual patients. By analyzing genetic data, AI algorithms can predict how patients respond to various treatments, allowing healthcare providers to choose the most effective therapy. This approach is advantageous in conditions with known genetic influences, such as certain cancers and rare genetic disorders.
- AI in Oncology for Customized Cancer Treatment Plans:
In oncology, AI develops personalized treatment plans based on tumor genetics and individual patient profiles. AI systems analyze data from various sources, including genetic sequencing and imaging, to identify the most effective treatment strategies for each cancer patient. This personalized approach improves treatment outcomes and reduces the likelihood of adverse reactions to therapy.
AI in Drug Discovery and Development
- Accelerating the Pace of New Drug Discovery:
AI dramatically accelerates the drug process, which traditionally takes years and requires significant investment. AI algorithms can quickly analyze vast chemical compounds and biological data databases to identify potential drug candidates. This process speeds up discovery and helps pinpoint more effective and safer drug candidates at an early stage.
- Enhancing the Accuracy of Clinical Trials:
In clinical trials, AI is used to improve patient selection and monitoring, thereby enhancing the accuracy and efficiency of trials. AI algorithms analyze patient data to identify the most suitable trial candidates, predict potential adverse reactions, and monitor patient responses. This targeted approach ensures that clinical trials are more efficient and have a higher probability of success.
Robotic Surgery and Rehabilitation
- Advancements in Robotic-Assisted Surgery:
Robotic surgery, enhanced by AI, has become increasingly prevalent in various surgical fields. AI-enhanced automated systems provide surgeons with enhanced precision, flexibility, and control, leading to less invasive procedures, reduced patient recovery times, and improved surgical outcomes. These systems can analyze data from pre-operative medical records to guide surgeons during procedures, optimizing surgical approaches and reducing complications.
- AI in Physical Therapy and Rehabilitation:
AI also transforms physical therapy and rehabilitation. Through the use of AI-powered devices and applications, patients receive personalized rehabilitation programs. These programs adjust based on real-time patient performance and feedback, ensuring patients receive the most effective treatment. Additionally, AI-enabled wearables and sensors provide continuous monitoring and feedback, aiding faster and more effective recovery.
Ethical and Legal Considerations
Privacy and Data Security
AI in healthcare necessitates collecting and analyzing large volumes of patient data. Ensuring the secure handling of this data is paramount to protect patient privacy; this involves implementing robust cybersecurity measures to safeguard against data breaches and unauthorized access. Additionally, healthcare providers must adhere to HIPAA (Health Insurance Portability and Accountability Act) regulations in the United States, which sets standards for patient data protection.
Maintaining patient confidentiality is crucial alongside data security. Patients must be aware of their data usage and provide consent, mainly when used for AI training. Stakeholders should establish transparent policies and procedures to ensure patients know their rights and the extent of data usage.
Liability and Accountability
One of the significant legal challenges in AI healthcare is determining liability when AI-assisted diagnoses or treatments go wrong. Establishing clear guidelines on the legal implications of AI decisions is essential; this includes delineating the responsibility between AI developers, healthcare providers, and the AI system.
While AI systems can significantly enhance healthcare delivery, the importance of human oversight cannot be understated. Maintaining a balance where AI supports, rather than replaces, human decision-making in healthcare is essential. Ensuring that healthcare professionals remain in the loop in all AI-assisted processes is crucial for maintaining accountability and making informed decisions.
Accessibility and Fairness
There is a risk that AI technologies in healthcare might widen existing health disparities if they are not made accessible to all segments of society. Efforts must ensure equitable access to AI-driven healthcare solutions, irrespective of the patient’s geographic location, economic status, or cultural background.
AI systems are only as unbiased as their training data. If the training data is skewed or not representative of the diverse patient population, there is a risk of algorithmic bias. This bias could lead to misdiagnoses or inappropriate treatment recommendations for certain groups of patients. Continuous monitoring and updating of AI algorithms are required to ensure they remain fair and unbiased.
Success Stories in AI-Assisted Diagnosis and Treatment
AI systems have shown remarkable success in oncology, particularly in diagnosing and developing treatment plans for various cancers. For example, IBM’s Watson for Oncology has been used to assist in identifying treatment options for cancer patients by analyzing medical data against a vast database of oncology literature.
In cardiology, AI has been instrumental in the early detection of heart diseases. Algorithms can analyze echocardiograms more accurately and faster than human cardiologists, leading to early interventions.
AI has made significant strides in neurology, particularly in detecting neurological disorders like Alzheimer’s. AI systems can analyze brain imaging to see early signs of Alzheimer’s, even before clinical symptoms manifest.
Challenges and Lessons Learned from Real-World Implementations
- Data Privacy and Security: Implementing AI in healthcare has highlighted data privacy and security challenges. Instances of data breaches have underscored the need for more robust cybersecurity measures and more stringent data handling protocols.
- Integration into Clinical Workflow: Another challenge has been the integration of AI into existing clinical workflows. AI tools must be user-friendly and seamlessly integrated into the healthcare system without disrupting existing practices.
- Managing Expectations: Managing expectations regarding what AI can and cannot do has been crucial. Understanding AI’s limitations helps prevent over-reliance on these systems and underscores the importance of human oversight.
Conclusion
Integrating Artificial Intelligence in healthcare represents a significant leap forward in medical science, offering groundbreaking advancements in diagnosis, treatment planning, and patient care. While AI has shown immense potential in enhancing healthcare services’ accuracy, efficiency, and personalization, it also presents unique ethical, legal, and practical challenges.
The success stories and lessons learned from real-world applications underscore the transformative impact of AI, yet they also highlight the need for careful consideration of privacy, security, and fairness. As we look to the future, the symbiotic relationship between AI and healthcare professionals will continue to evolve, driven by ongoing research and technological advancements.
By addressing these challenges and harnessing the power of AI responsibly, we stand on the cusp of a new era in medicine — one that promises more precise, effective, and accessible healthcare for all.
How AI Diagnosis and Treatment Applications Boost the Quality of Healthcare – Report
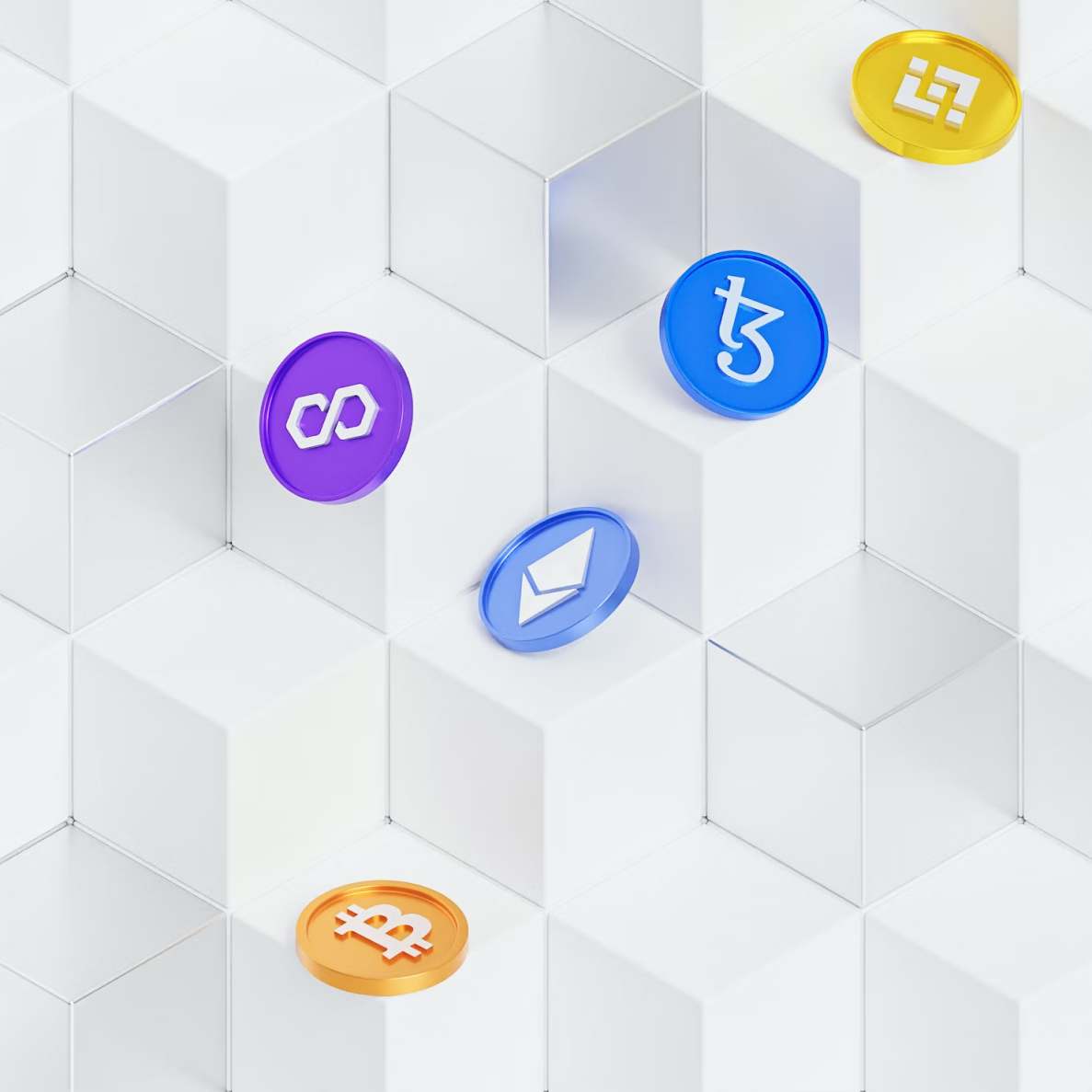
In recent years, the intersection of artificial intelligence (AI) and healthcare has become an area of intense research and transformative potential. AI diagnosis, which uses a wide range of technologies capable of performing tasks that typically require human intelligence, is pivotal in reshaping various treatment methods. This article aims to explore the multifaceted applications of AI in healthcare, mainly focusing on its contributions to diagnosing diseases and formulating treatment plans.
The evolution of AI in medical contexts has been both rapid and revolutionary. From its nascent stages of simple data analysis to its current capabilities in complex decision-making, AI has increasingly become an integral component of modern healthcare systems. Its integration into medical practices has significantly improved patient care’s accuracy, efficiency, and personalization.
Background
The journey of AI in healthcare began in the late 20th century, marked by pioneering efforts to integrate computational power into medical practices. The earliest applications were rudimentary by today’s standards, primarily focused on database management and basic patient record systems. In the 1960s and 70s, more sophisticated systems like the Dendral project, which interpreted organic chemical mass spectra, and MYCIN, developed at Stanford University for diagnosing blood infections and recommending antibiotics, laid the groundwork for AI in medical diagnostics. These systems used rule-based methods to mimic human decision-making, offering early glimpses into AI’s potential in healthcare.
Milestones in AI Development for Healthcare Purposes
- Rule-Based Expert Systems: In the 1980s, expert systems like CADUCEUS and INTERNIST-I demonstrated AI’s potential in capturing medical knowledge and diagnostic reasoning. Though limited by the technology of their time, these systems were significant in showcasing how AI could store and utilize vast medical knowledge.
- Advent of Machine Learning: The 1990s and 2000s witnessed a pivotal shift with the introduction of machine learning (ML). Algorithms could now learn from data, improving their accuracy and utility over time. This era saw the development of neural networks and decision trees, crucial in analyzing complex medical data.
- Genomics and Personalized Medicine: Completing the Human Genome Project in the early 2000s opened new avenues for AI in genomics. AI’s ability to analyze large genetic datasets has been instrumental in the growth of personalized medicine, tailoring healthcare based on individual genetic profiles.
- Deep Learning Revolution: The 2010s marked the deep learning revolution, characterized by the advent of algorithms capable of feature learning from large datasets. This breakthrough led to significant advancements in medical imaging, drug discovery, and predictive analytics.
Integration of AI with Traditional Medical Practices
The integration of AI into healthcare has required close collaboration with medical professionals. AI systems complement the expertise of doctors, nurses, and other healthcare workers. This coordination is crucial for fine-tuning AI applications for practical, real-world medical scenarios.
Alongside technological advancements, there has been a growing emphasis on developing ethical and regulatory frameworks to govern the use of AI in healthcare. These frameworks are essential to ensure patient safety, data privacy, and the responsible use of AI.
The integration process also involves educating and training healthcare professionals in AI technologies. This education ensures medical practitioners can work alongside AI tools, understanding their capabilities and limitations.
AI has played a significant role in enhancing patient engagement and the rise of telemedicine. Tools such as chatbots for preliminary diagnosis and mobile apps for health monitoring have brought healthcare closer to patients, making it more accessible and personalized.
AI in Diagnosis
Image-based Diagnostics
- Radiology and Imaging Analysis:
AI has revolutionized radiology by enhancing the interpretation of medical images such as X-rays, CT scans, and MRIs. Machine learning algorithms, especially deep learning models, can now accurately detect anomalies like tumors, fractures, and other pathological changes. For instance, AI systems can identify patterns in lung imaging indicative of diseases such as pneumonia or tuberculosis, often at early stages that may be challenging for the human eye to discern.
- Dermatology and Skin Disease Recognition:
In dermatology, AI algorithms have shown proficiency in recognizing skin lesions and differentiating between benign and malignant conditions. Utilizing vast databases of skin images, these systems help dermatologists detect skin cancers like melanoma early, facilitating timely treatment. AI tools can also assist patients in monitoring skin changes, allowing for early consultation and diagnosis.
- Ophthalmology and Retinal Image Interpretation:
AI has made significant strides in ophthalmology, particularly in analyzing retinal images. Algorithms have been developed to detect diabetic retinopathy, glaucoma, and age-related macular degeneration, among the leading causes of blindness. These AI systems provide eye care professionals with powerful tools to identify and treat these conditions much earlier than possible.
Genetic and Laboratory Data Analysis
- Predictive Modeling in Genomics:
AI’s role in genomics involves analyzing vast genetic data sets to predict disease risk and treatment outcomes. By employing techniques like machine learning, AI can identify genetic markers associated with diseases, thereby aiding in early detection and personalized treatment plans. This approach is particularly impactful in oncogenetics, where AI helps understand the genetic basis of cancers.
- Automation in Laboratory Result Interpretation:
AI has automated and improved the accuracy of laboratory result interpretation. Algorithms analyze blood tests, urinalysis, and other laboratory data, providing quicker and more accurate diagnoses. This automation reduces the workload on laboratory personnel and minimizes human error, leading to better patient outcomes.
AI in Diagnostic Decision Support
- Case Studies of AI-assisted Diagnostics:
Various case studies demonstrate the successful implementation of AI in diagnostic processes. For example, hospitals have employed AI systems to diagnose neurological disorders, cardiovascular diseases, and various cancers, often revealing subtleties missed in traditional analyses.
- Improving Accuracy and Reducing Diagnostic Errors:
AI contributes significantly to reducing diagnostic errors, a significant healthcare concern. By providing a second, unbiased opinion, AI systems can identify discrepancies in diagnoses and suggest alternative possibilities. This dual-check system ensures accuracy and can be life-saving in critical care and emergency medicine.
- Ethical Considerations and Limitations:
Despite the benefits, the use of AI in diagnosis raises ethical questions, particularly regarding data privacy, consent, and the potential for algorithmic bias. Ensuring that AI systems are from diverse datasets is crucial to avoid biases that could lead to misdiagnosis. Furthermore, the reliance on AI should not diminish the role of human judgment in healthcare; instead, we should view AI as a tool that augments the expertise of medical professionals.
AI in Treatment Planning and Management
Personalized Medicine
- Tailoring Treatment Based on Genetic Information:
AI’s role in personalized medicine is transformative, especially in utilizing genetic information to tailor treatments to individual patients. By analyzing genetic data, AI algorithms can predict how patients respond to various treatments, allowing healthcare providers to choose the most effective therapy. This approach is advantageous in conditions with known genetic influences, such as certain cancers and rare genetic disorders.
- AI in Oncology for Customized Cancer Treatment Plans:
In oncology, AI develops personalized treatment plans based on tumor genetics and individual patient profiles. AI systems analyze data from various sources, including genetic sequencing and imaging, to identify the most effective treatment strategies for each cancer patient. This personalized approach improves treatment outcomes and reduces the likelihood of adverse reactions to therapy.
AI in Drug Discovery and Development
- Accelerating the Pace of New Drug Discovery:
AI dramatically accelerates the drug process, which traditionally takes years and requires significant investment. AI algorithms can quickly analyze vast chemical compounds and biological data databases to identify potential drug candidates. This process speeds up discovery and helps pinpoint more effective and safer drug candidates at an early stage.
- Enhancing the Accuracy of Clinical Trials:
In clinical trials, AI is used to improve patient selection and monitoring, thereby enhancing the accuracy and efficiency of trials. AI algorithms analyze patient data to identify the most suitable trial candidates, predict potential adverse reactions, and monitor patient responses. This targeted approach ensures that clinical trials are more efficient and have a higher probability of success.
Robotic Surgery and Rehabilitation
- Advancements in Robotic-Assisted Surgery:
Robotic surgery, enhanced by AI, has become increasingly prevalent in various surgical fields. AI-enhanced automated systems provide surgeons with enhanced precision, flexibility, and control, leading to less invasive procedures, reduced patient recovery times, and improved surgical outcomes. These systems can analyze data from pre-operative medical records to guide surgeons during procedures, optimizing surgical approaches and reducing complications.
- AI in Physical Therapy and Rehabilitation:
AI also transforms physical therapy and rehabilitation. Through the use of AI-powered devices and applications, patients receive personalized rehabilitation programs. These programs adjust based on real-time patient performance and feedback, ensuring patients receive the most effective treatment. Additionally, AI-enabled wearables and sensors provide continuous monitoring and feedback, aiding faster and more effective recovery.
Ethical and Legal Considerations
Privacy and Data Security
AI in healthcare necessitates collecting and analyzing large volumes of patient data. Ensuring the secure handling of this data is paramount to protect patient privacy; this involves implementing robust cybersecurity measures to safeguard against data breaches and unauthorized access. Additionally, healthcare providers must adhere to HIPAA (Health Insurance Portability and Accountability Act) regulations in the United States, which sets standards for patient data protection.
Maintaining patient confidentiality is crucial alongside data security. Patients must be aware of their data usage and provide consent, mainly when used for AI training. Stakeholders should establish transparent policies and procedures to ensure patients know their rights and the extent of data usage.
Liability and Accountability
One of the significant legal challenges in AI healthcare is determining liability when AI-assisted diagnoses or treatments go wrong. Establishing clear guidelines on the legal implications of AI decisions is essential; this includes delineating the responsibility between AI developers, healthcare providers, and the AI system.
While AI systems can significantly enhance healthcare delivery, the importance of human oversight cannot be understated. Maintaining a balance where AI supports, rather than replaces, human decision-making in healthcare is essential. Ensuring that healthcare professionals remain in the loop in all AI-assisted processes is crucial for maintaining accountability and making informed decisions.
Accessibility and Fairness
There is a risk that AI technologies in healthcare might widen existing health disparities if they are not made accessible to all segments of society. Efforts must ensure equitable access to AI-driven healthcare solutions, irrespective of the patient’s geographic location, economic status, or cultural background.
AI systems are only as unbiased as their training data. If the training data is skewed or not representative of the diverse patient population, there is a risk of algorithmic bias. This bias could lead to misdiagnoses or inappropriate treatment recommendations for certain groups of patients. Continuous monitoring and updating of AI algorithms are required to ensure they remain fair and unbiased.
Success Stories in AI-Assisted Diagnosis and Treatment
AI systems have shown remarkable success in oncology, particularly in diagnosing and developing treatment plans for various cancers. For example, IBM’s Watson for Oncology has been used to assist in identifying treatment options for cancer patients by analyzing medical data against a vast database of oncology literature.
In cardiology, AI has been instrumental in the early detection of heart diseases. Algorithms can analyze echocardiograms more accurately and faster than human cardiologists, leading to early interventions.
AI has made significant strides in neurology, particularly in detecting neurological disorders like Alzheimer’s. AI systems can analyze brain imaging to see early signs of Alzheimer’s, even before clinical symptoms manifest.
Challenges and Lessons Learned from Real-World Implementations
- Data Privacy and Security: Implementing AI in healthcare has highlighted data privacy and security challenges. Instances of data breaches have underscored the need for more robust cybersecurity measures and more stringent data handling protocols.
- Integration into Clinical Workflow: Another challenge has been the integration of AI into existing clinical workflows. AI tools must be user-friendly and seamlessly integrated into the healthcare system without disrupting existing practices.
- Managing Expectations: Managing expectations regarding what AI can and cannot do has been crucial. Understanding AI’s limitations helps prevent over-reliance on these systems and underscores the importance of human oversight.
Conclusion
Integrating Artificial Intelligence in healthcare represents a significant leap forward in medical science, offering groundbreaking advancements in diagnosis, treatment planning, and patient care. While AI has shown immense potential in enhancing healthcare services’ accuracy, efficiency, and personalization, it also presents unique ethical, legal, and practical challenges.
The success stories and lessons learned from real-world applications underscore the transformative impact of AI, yet they also highlight the need for careful consideration of privacy, security, and fairness. As we look to the future, the symbiotic relationship between AI and healthcare professionals will continue to evolve, driven by ongoing research and technological advancements.
By addressing these challenges and harnessing the power of AI responsibly, we stand on the cusp of a new era in medicine — one that promises more precise, effective, and accessible healthcare for all.