Machine Learning Tool Offers Hope in Diagnosing Cancer of Unknown Primary
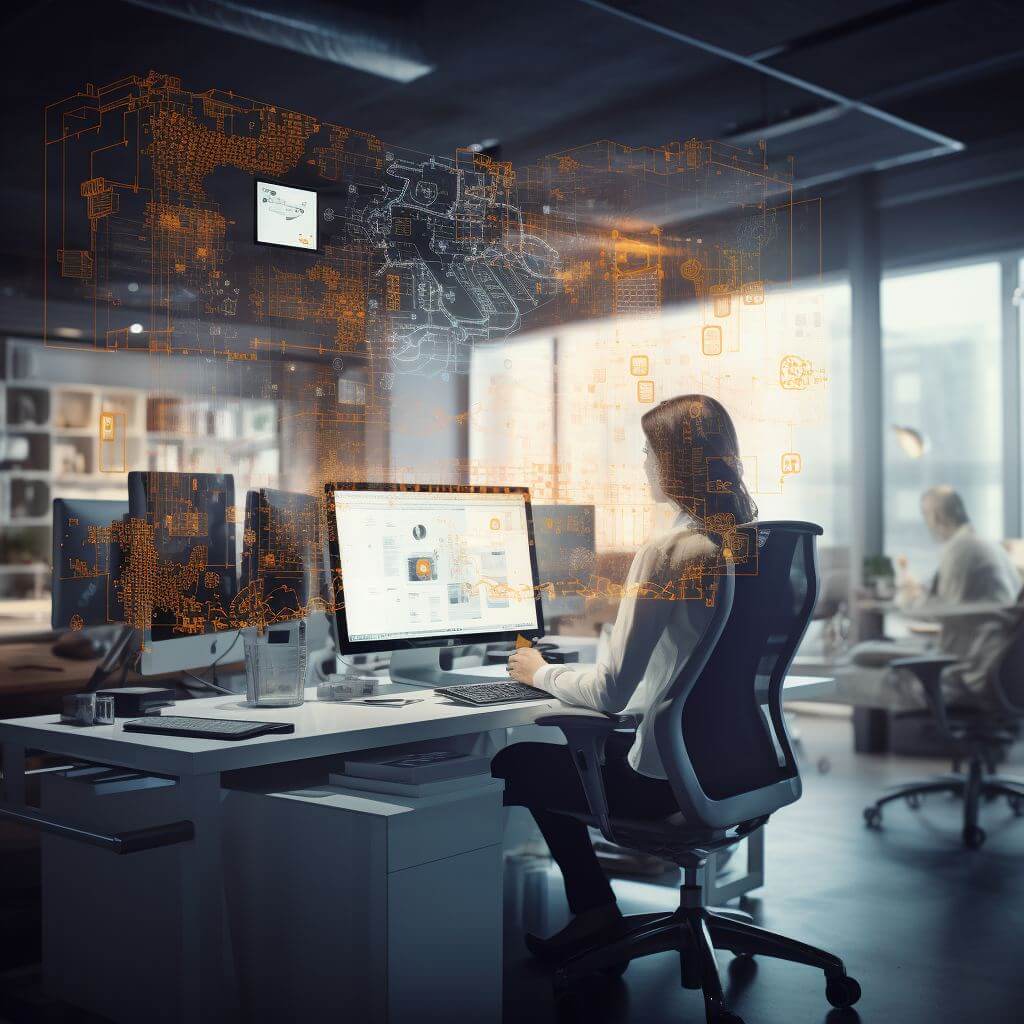
Most cancers originate in a specific body part, making it crucial for physicians to pinpoint the source to treat patients effectively. However, in three to five percent of cases, the standard diagnostic workup fails to identify the primary site, leading to a grim prognosis for those with this condition known as Cancer of Unknown Primary (CUP). Often, doctors resort to broad treatments that are less effective and accompanied by more side effects than targeted therapies. As a result, CUP patients face rapid disease progression, with survival rates averaging only six to 16 months.
Introducing OncoNPC: A machine learning solution
Intae Moon, a PhD student at the Massachusetts Institute of Technology (MIT) and the Dana-Farber Cancer Institute, sought to revolutionize the diagnosis of CUP’s primary site. While Next Generation Sequencing (NGS) can identify tumor mutations and assist in determining cancer types, the vast amount of mutation data generated can overwhelm physicians during the initial diagnosis. Typically, NGS is used only after cancer type identification to pinpoint specific mutations for targeted therapies. However, NGS had not been extensively explored for CUP’s clinical diagnosis and prognosis. Moon and colleagues developed a machine learning model known as OncoNPC (Oncology NGS-based Primary Cancer-type Classifier) to bridge this gap. This innovative approach aims to analyze complex mutation data efficiently.
Unleashing the power of machine learning
Published in Nature Medicine, the study harnesses the capabilities of the XGBoost algorithm to scrutinize DNA regions for patterns of genetic mutations most strongly associated with various cancer types. Leveraging NGS data from 36,445 tumor samples with known primary cancers, the researchers employed OncoNPC to identify genetic mutations, copy number alterations, and mutational signatures. The model also incorporated patient age and biological sex from electronic health records. By training OncoNPC on data from three different cancer centers across the US, the team linked specific genetic signatures with 22 different cancer types. After training, they evaluated OncoNPC’s performance on previously excluded data, achieving an impressive accuracy rate.
A beacon of hope for CUP patients
Applying OncoNPC to tumors from 971 CUP patients treated at the Dana-Farber Cancer Institute, the researchers successfully classified 41.2 percent of CUP tumors with high confidence. Furthermore, they identified the genetic features most relevant for identifying each cancer type, providing invaluable clinical and biological insights into the enigmatic nature of CUP tumors.
Given that the primary tumor sites were unknown in these cases, the model’s accuracy was validated by comparing results against the patients’ NGS data to identify any genetic predisposition for a specific cancer type. Encouragingly, the model’s predictions closely aligned with the cancer type indicated most strongly by these inherited mutations.
Enhancing patient survival through precision treatment
To assess the impact of treating patients according to OncoNPC predictions on their survival, the team conducted a retrospective analysis of 158 CUP patients who received their initial treatment at Dana-Farber. Certified oncologists reviewed patient charts to determine if treatments aligned with OncoNPC’s cancer-type predictions. The results were promising, showing that CUP patients whose first palliative treatment matched OncoNPC predictions experienced significantly improved survival rates compared to those whose treatments did not align.
Prospects and expanding horizons
The researchers have ambitious plans for OncoNPC’s future. While the model demonstrated decent performance for patients of various ethnic backgrounds, further research is needed to confirm its effectiveness across diverse patients. Additionally, OncoNPC focuses on the 22 most common cancer types and may not identify the source if the CUP originates from a less common site.
However, the potential impact of OncoNPC in the medical field is undeniable. Hospitals utilizing NGS tumor sequencing may incorporate it as an additional source of information for oncologists, paving the way for more precise and effective cancer treatment decisions. This breakthrough, rooted in routine clinical testing data, offers hope for many CUP patients, whose prognosis has long been dire.
In the relentless battle against cancer, the emergence of OncoNPC represents a ray of hope for patients with Cancer of Unknown Primary. With its impressive accuracy in identifying cancer types and the potential to improve patient survival through precision treatment, this machine-learning tool could revolutionize how physicians diagnose and manage this challenging condition. While there are challenges to overcome and further research to be done, OncoNPC’s potential to make a difference in the lives of CUP patients is both promising and groundbreaking.
Machine Learning Tool Offers Hope in Diagnosing Cancer of Unknown Primary
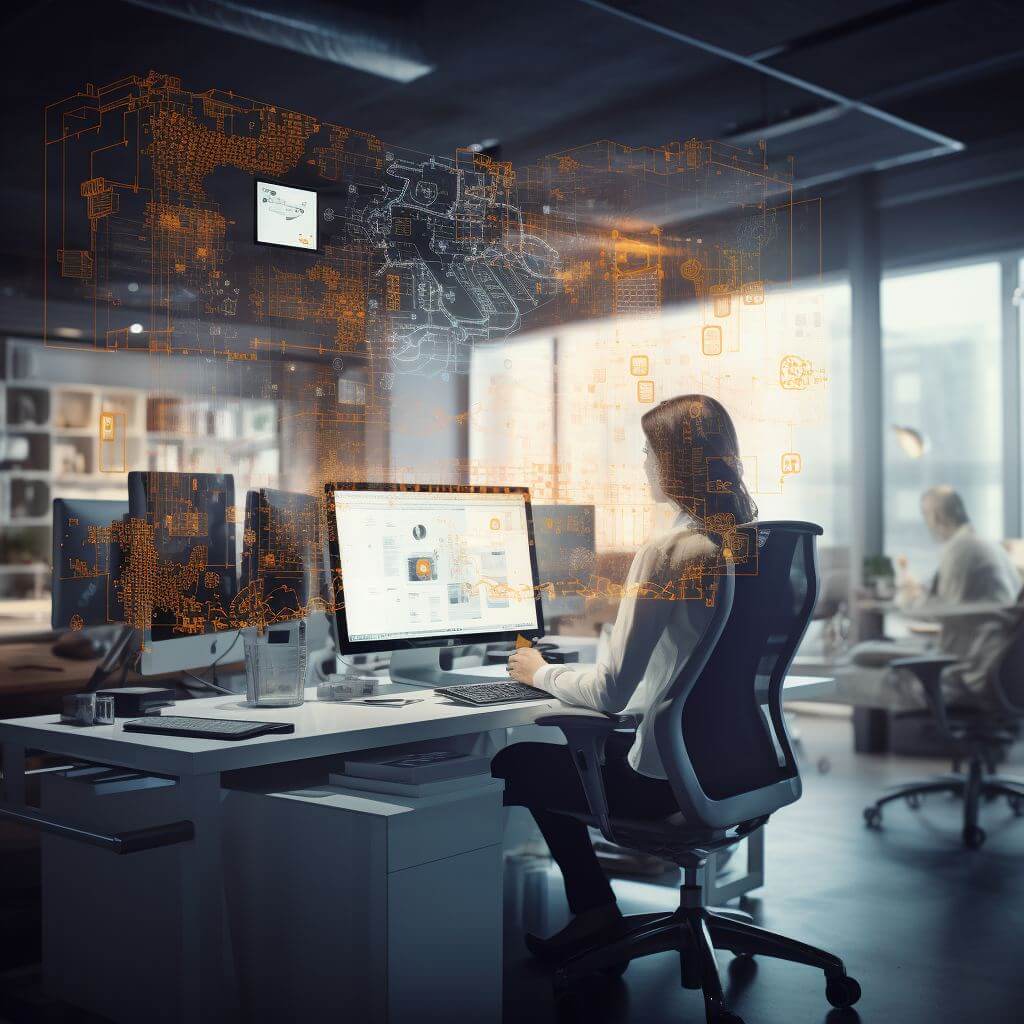
Most cancers originate in a specific body part, making it crucial for physicians to pinpoint the source to treat patients effectively. However, in three to five percent of cases, the standard diagnostic workup fails to identify the primary site, leading to a grim prognosis for those with this condition known as Cancer of Unknown Primary (CUP). Often, doctors resort to broad treatments that are less effective and accompanied by more side effects than targeted therapies. As a result, CUP patients face rapid disease progression, with survival rates averaging only six to 16 months.
Introducing OncoNPC: A machine learning solution
Intae Moon, a PhD student at the Massachusetts Institute of Technology (MIT) and the Dana-Farber Cancer Institute, sought to revolutionize the diagnosis of CUP’s primary site. While Next Generation Sequencing (NGS) can identify tumor mutations and assist in determining cancer types, the vast amount of mutation data generated can overwhelm physicians during the initial diagnosis. Typically, NGS is used only after cancer type identification to pinpoint specific mutations for targeted therapies. However, NGS had not been extensively explored for CUP’s clinical diagnosis and prognosis. Moon and colleagues developed a machine learning model known as OncoNPC (Oncology NGS-based Primary Cancer-type Classifier) to bridge this gap. This innovative approach aims to analyze complex mutation data efficiently.
Unleashing the power of machine learning
Published in Nature Medicine, the study harnesses the capabilities of the XGBoost algorithm to scrutinize DNA regions for patterns of genetic mutations most strongly associated with various cancer types. Leveraging NGS data from 36,445 tumor samples with known primary cancers, the researchers employed OncoNPC to identify genetic mutations, copy number alterations, and mutational signatures. The model also incorporated patient age and biological sex from electronic health records. By training OncoNPC on data from three different cancer centers across the US, the team linked specific genetic signatures with 22 different cancer types. After training, they evaluated OncoNPC’s performance on previously excluded data, achieving an impressive accuracy rate.
A beacon of hope for CUP patients
Applying OncoNPC to tumors from 971 CUP patients treated at the Dana-Farber Cancer Institute, the researchers successfully classified 41.2 percent of CUP tumors with high confidence. Furthermore, they identified the genetic features most relevant for identifying each cancer type, providing invaluable clinical and biological insights into the enigmatic nature of CUP tumors.
Given that the primary tumor sites were unknown in these cases, the model’s accuracy was validated by comparing results against the patients’ NGS data to identify any genetic predisposition for a specific cancer type. Encouragingly, the model’s predictions closely aligned with the cancer type indicated most strongly by these inherited mutations.
Enhancing patient survival through precision treatment
To assess the impact of treating patients according to OncoNPC predictions on their survival, the team conducted a retrospective analysis of 158 CUP patients who received their initial treatment at Dana-Farber. Certified oncologists reviewed patient charts to determine if treatments aligned with OncoNPC’s cancer-type predictions. The results were promising, showing that CUP patients whose first palliative treatment matched OncoNPC predictions experienced significantly improved survival rates compared to those whose treatments did not align.
Prospects and expanding horizons
The researchers have ambitious plans for OncoNPC’s future. While the model demonstrated decent performance for patients of various ethnic backgrounds, further research is needed to confirm its effectiveness across diverse patients. Additionally, OncoNPC focuses on the 22 most common cancer types and may not identify the source if the CUP originates from a less common site.
However, the potential impact of OncoNPC in the medical field is undeniable. Hospitals utilizing NGS tumor sequencing may incorporate it as an additional source of information for oncologists, paving the way for more precise and effective cancer treatment decisions. This breakthrough, rooted in routine clinical testing data, offers hope for many CUP patients, whose prognosis has long been dire.
In the relentless battle against cancer, the emergence of OncoNPC represents a ray of hope for patients with Cancer of Unknown Primary. With its impressive accuracy in identifying cancer types and the potential to improve patient survival through precision treatment, this machine-learning tool could revolutionize how physicians diagnose and manage this challenging condition. While there are challenges to overcome and further research to be done, OncoNPC’s potential to make a difference in the lives of CUP patients is both promising and groundbreaking.